Understanding the biomolecular world within us, and how complex networks of molecules interact within our cells, is a crucial starting point for understanding and treating disease with rational drug design.
To move this understanding forwards, together with Google DeepMind we have developed AlphaFold 3, our breakthrough artificial intelligence (AI) model that provides an accurate atomic-level view of the structure of biomolecular systems. This builds upon Google DeepMind’s foundational work of predicting the structure of proteins with AlphaFold 2, to now include how multiple proteins, DNA, RNA, and small molecule ligands come together and interact, as well as predicting the structural impact of post-translational modifications and ions on these molecular systems. This model is a powerful unified framework for structure prediction, encompassing unprecedented breadth and accuracy.
This breakthrough opens up exciting possibilities for drug discovery, allowing us to rationally develop therapeutics against targets that were previously difficult or deemed intractable to modulate.
AlphaFold 3 and the research that led to this breakthrough
AlphaFold 3 is an AI model that allows a scientist to input a description of a biomolecular complex that they are interested in, and predicts the 3D structure of that biomolecular complex.
The input biomolecular system can consist of a collection of multiple proteins, nucleic acids (DNA and RNA), as well as small molecule ligands and ions. These inputs are processed by AlphaFold 3, which is a generative model composed of a neural network architecture that builds upon a custom Transformer with triangular attention, and uses a diffusion process to generate individual 3D coordinates of every atom in the input-specified system.
Building this technology required a huge amount of innovation and research, developed with our colleagues at Google DeepMind. The model is trained on the world’s molecular structural data contained within the Protein Data Bank, and is able to process over 99% of all known biomolecular complexes contained there. The capabilities and accuracy of this model have been extensively evaluated across a wide range of test cases including many completely novel systems and molecular interfaces. From these evaluations, we’ve seen state-of-the-art accuracy across nearly all structural areas, including doubling the accuracy for some important interfaces, whilst generalising even to novel interfaces that are not seen during training, such as the examples depicted in this blog post.
We’re really excited to share the hard work of our teams, with more details of the model and results detailed in our Nature paper.
We’re also looking forward to seeing how scientists will use the AFServer released today to generate molecular complexes for non-commercial academic research to accelerate our understanding of biology.
Three examples that show how AF3 allows us to fold many proteins with their respective ligands, and to rationalise their mechanism of action. Ground truth structures are shown in white.

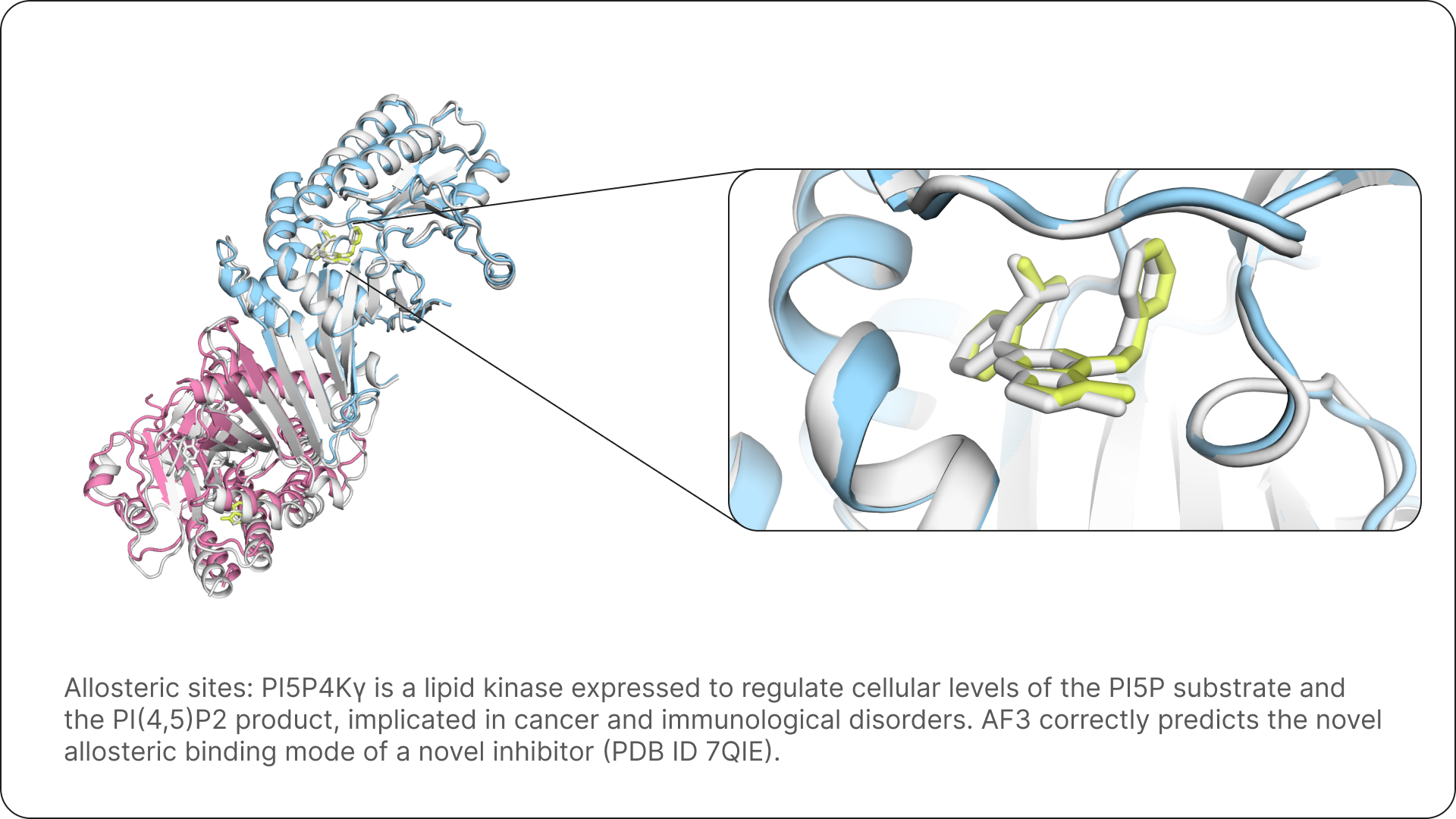
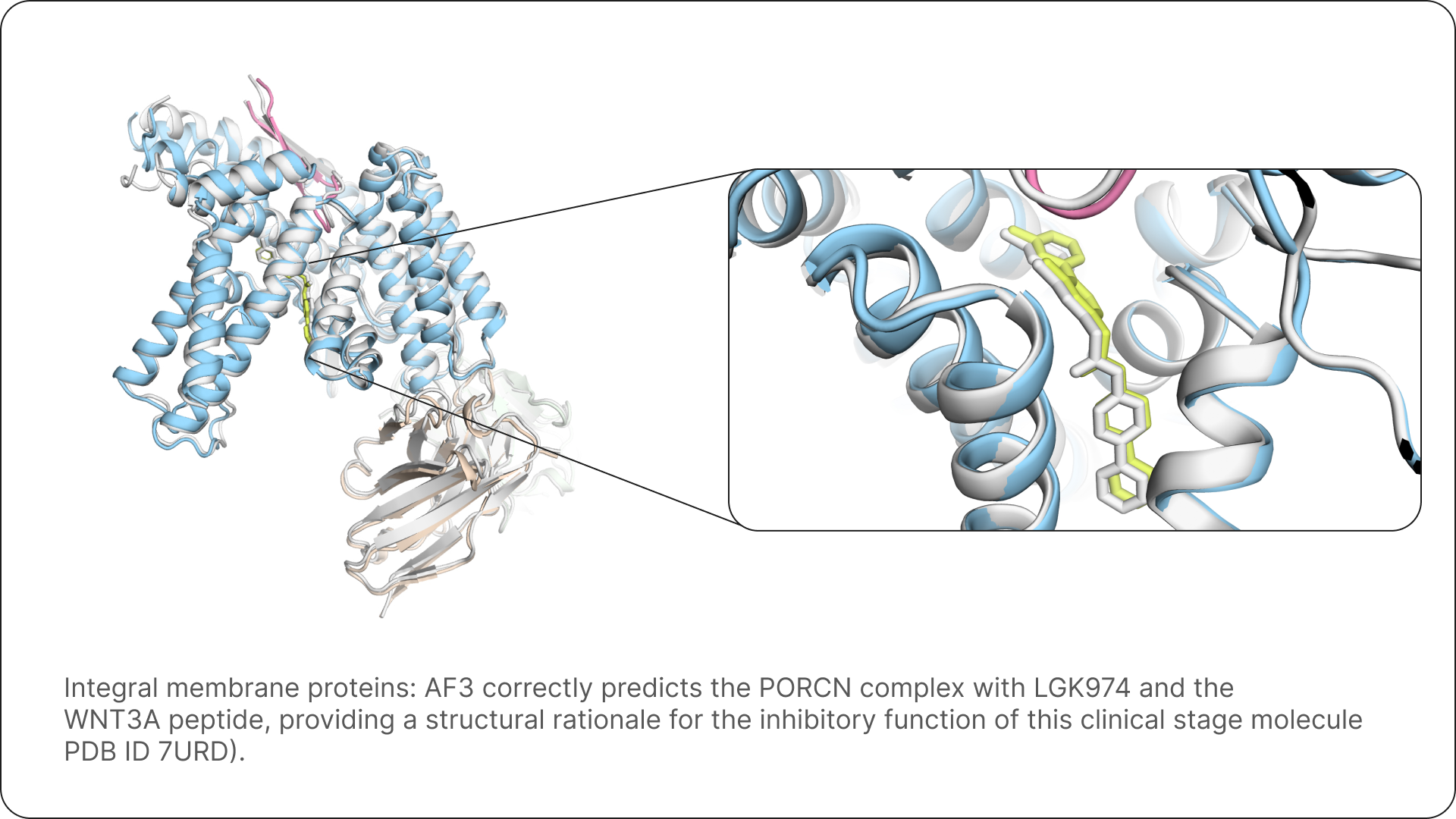
The promise of AlphaFold 3 for science and drug design
For Isomorphic Labs, AlphaFold 3 equips our drug designers with the ability to quickly and accurately predict the structure of complexes that have never been characterised before, giving us a fundamental tool that allows us to take novel approaches to drug design.
We can now create and test hypotheses at the atomic level, and produce highly accurate structure predictions within seconds, standing in stark contrast to the months, or even years, required to experimentally determine answers to similar questions.
Already, we’re using AlphaFold 3 day-to-day. Our scientists have seen:
- That designing small molecules against AlphaFold 3’s structural predictions helps create designs that bind effectively to a target protein.
- The improved structural accuracy of protein-protein interfaces with AlphaFold 3 opens up the possibility of designing for new treatment modalities such as antibodies or other therapeutic proteins.
- A richer understanding of a novel target can be achieved by looking at the structure of targets in their full biological context, in complex with other protein binding partners, DNA, RNA, and ligand cofactors. We believe that this broader understanding of the context within which drug targets operate will translate into more effective drugs in the clinic.
To demonstrate the potential for rational structure-based drug design with AlphaFold 3, we examined TIM-3, an immune checkpoint protein identified as a potential target for cancer immunotherapy following a 2021 publication.
The study focused on the discovery and design of small molecules capable of binding TIM-3 with high affinity. The research group experimentally solved three ligand-bound crystal structures to rationalise the structure-activity relationship observed in their work. To our knowledge, no small molecule-bound crystal structures of TIM-3 existed in the Protein Data Bank prior to this paper, and these structures were not in the training set of AlphaFold 3.
Crucially, the authors found those ligands bound to a previously uncharacterised pocket.
We evaluated this system with AlphaFold 3, by creating three predictions corresponding to the three published crystal structures. We used the raw sequence of the protein, and the SMILES representation of each ligand, without giving AlphaFold 3 any additional information about the pose, structure, or pocket. Excitingly, the predicted structures were in agreement with the published experimental structures. The pocket discovered by the study was also found by AlphaFold 3. Furthermore, the predicted binding modes were almost identical to the ground truth structures, and the ligand-free prediction we predicted for reference showed a very different pocket conformation, which was flat and open. This difference between the predicted protein structure with and without the ligand demonstrates the ability of AlphaFold 3 to change the structure of the protein based on the presence of other molecules in a context dependent manner.
Through this example, AlphaFold 3 demonstrated it could accurately characterise the progression of a drug molecule design structurally.
Looking ahead
We’ve already deployed our frontier version of AlphaFold 3 in our own internal pipeline of projects, and in our partnerships with pharmaceutical companies.
But AlphaFold 3 is one of the many AI-powered breakthroughs we’re working on which are needed to transform drug discovery - structural understanding is just a part of the picture. We’re combining AlphaFold 3 with our other proprietary AI models in our platform that help us understand more about the properties, function, and dynamics of molecular systems. And as we learn more about the molecular machines within us, with more structural and biological context, we can use this understanding to identify novel targets for drug design, as well as to approach existing targets with novel therapeutic mechanisms.
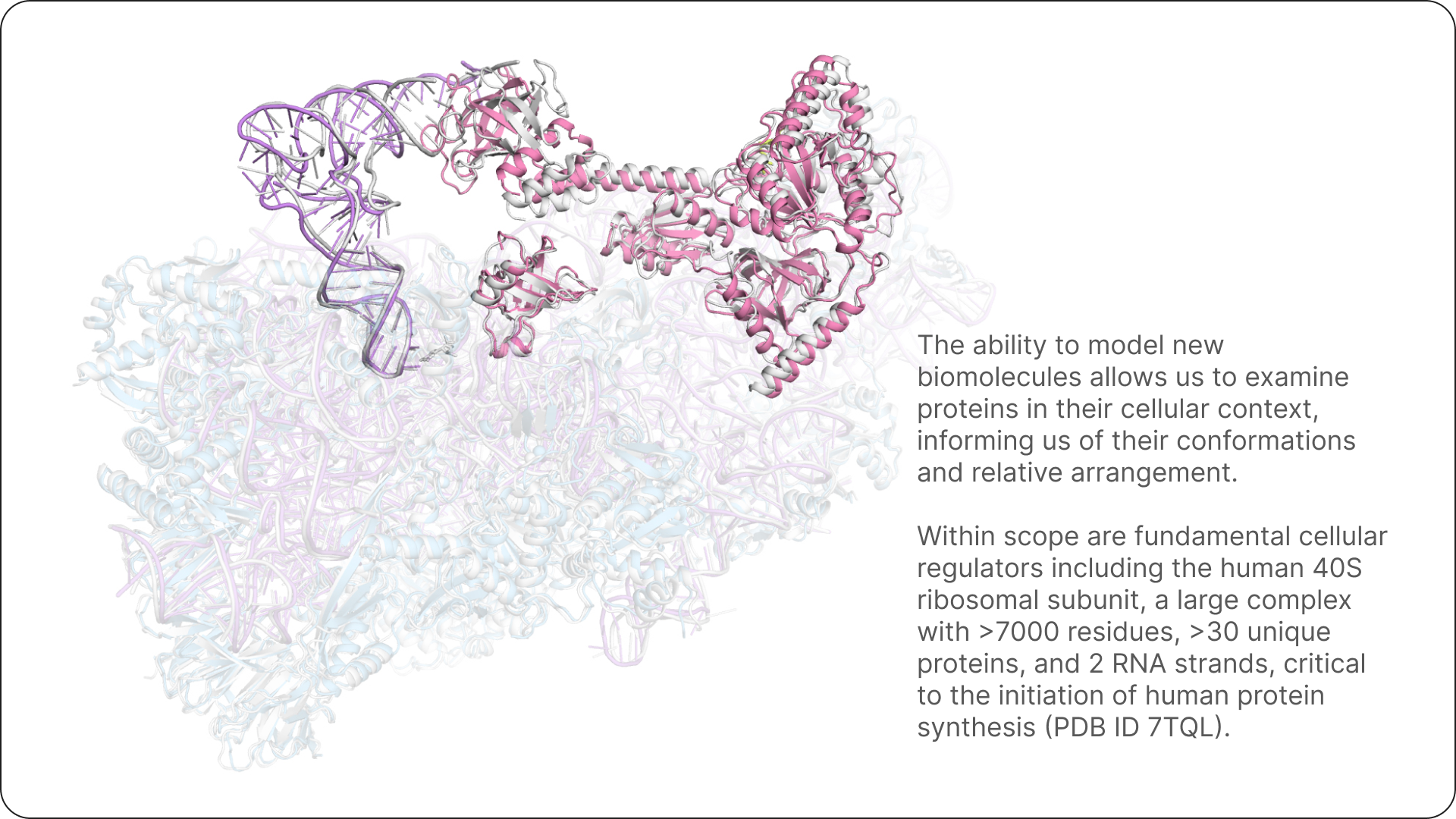
We’ll continue to be heads down in research, tackling the next frontier of fundamental modelling questions in chemistry and biology from first principles with AI. Bringing these together will help change the way we design the next generation of therapeutics, and unlock new biology.
While this is an important moment for AI-powered biological research, the potential for AI to accelerate outcomes for digital biology are limitless. Further development of our AI research models will deepen our understanding of human biology and the building blocks of life to reach our ultimate goal - harnessing the power and pace of AI to reimagine the entire drug discovery process.
Read more:
AlphaFold 3 predicts the structure and interactions of all life's molecules